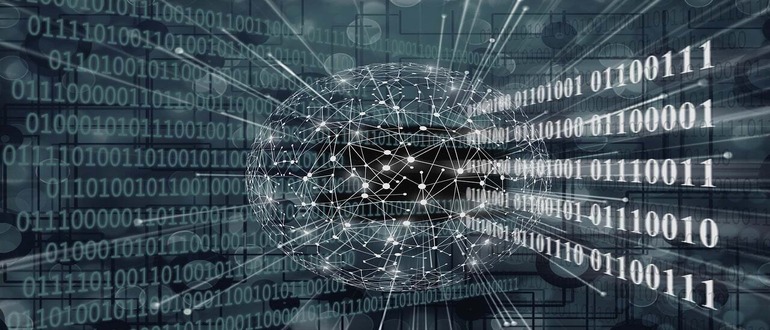
Picture this: You’re scrolling through your favorite video streaming platform, not sure what you’re in the mood for, and suddenly, the perfect recommendation pops up. It’s a film you’d never have chosen on your own—perhaps even a genre you rarely watch—but somehow, it feels like it was meant for you. This isn’t just another algorithm at work. It’s what can be described as AI-powered serendipity, a phenomenon where advanced technology seemingly reads your mind and surprises you with exactly what you didn’t know you needed.
At its core, AI-powered serendipity is a blend of personalization, prediction, and subtle discovery. It combines the science of machine learning (ML) with the art of creating moments of unexpected user delight. But how does it do it? And why is it emerging as the holy grail for businesses looking to captivate their customers? Let’s jump right into it.
The Art and Science of Serendipity
For years, algorithms have operated in a highly deterministic fashion: if you like X, then you’ll probably like Y. And this is usually covered under traditional affinity analysis or correlation. This approach, more popularly known as collaborative filtering, has powered everything from movie suggestions on Netflix to product recommendations on Amazon–for the most part. It’s effective—but is it everything we can get?
Serendipity, on the other hand, introduces an element of the unexpected. It’s not just about confirming your known preferences; it’s stemming from interpreting user nuances and is all about nudging you toward something adjacent, novel, or entirely surprising—while still being relevant.
Achieving this blend requires going beyond traditional recommendation engines. It involves multimodal machine learning models capable of understanding and integrating diverse data types, such as textual data, like your search queries, reviews, and descriptions; visual data as in images or video thumbnails you hover over or engage with; and behavioral data which is scrolling, clicks, and pauses while playing a video.
By analyzing these varied inputs, AI can map out your explicit preferences while uncovering implicit signals—preferences you may not even be aware of yourself. This is where serendipity thrives: in the overlap between what you know you like and what you’re open to discovering that is a joyful revelation.
How Machine Learning Powers Serendipity
At a technical level, AI-powered serendipity relies on advancements in neural networks and representation learning. Here’s how it broadly works:
Building Rich User Profiles with Multimodal Data
Traditional recommendation engines rely heavily on sparse data — like explicit ratings or purchase histories. Advanced AI-powered systems, however, use deep learning models to analyze multimodal data sources. Transformer-based models like BERT or GPT analyze textual inputs to understand user sentiment and intent; Convolutional Neural Networks (CNNs) process images and videos to identify patterns in visual preferences; Sequence models like RNNs track user behaviors over time, creating dynamic profiles that evolve with each interaction.
For example, if you frequently pause on videos with vibrant cinematography of the outdoors or engage with content featuring a particular actor, these signals are captured and integrated into your profile where you could be cueing you either like nature or that specific actor.
Exploring Latent Preferences
AI doesn’t just predict what you like—it predicts the why behind your choices. Using latent factor analysis, machine learning models identify hidden dimensions of user behavior. Let’s consider Spotify’s AI-driven Discover Weekly playlist. The system doesn’t just know you like acoustic folk music; it analyzes intricate attributes like tempo, mood, and instrumentation to surface tracks that align with your deeper preferences.
This same principle applies across industries, enabling more serendipitous discoveries in shopping, travel, and entertainment.
Balancing Familiarity and Novelty
A critical aspect of serendipity is achieving the right balance between familiarity and novelty. Too much novelty, and the recommendation feels irrelevant. Too much familiarity, and it becomes predictable.
To solve this, AI models use techniques like Reinforcement Learning (RL). RL algorithms optimize for long-term user satisfaction by introducing a degree of exploration into the recommendation process. This means occasionally suggesting something outside your typical patterns to gauge user reaction.
Another technique is hybrid filtering. By combining collaborative and content-based filtering, the system expands beyond your immediate preferences while still ensuring relevance.
For example, an AI system might notice your interest in true crime podcasts but recommend a dark comedy series based on similar thematic components. It’s a small and an obvious leap—but one that feels perfectly tailored.
Contextual Recommendations in Real Time
Modern AI doesn’t just recommend based on static data; it adapts to the context of each discussion or interaction. This involves context-aware recommendation models, which consider factors like time and location, and device usage. What you watch during a weekday lunch break may differ from your weekend or Friday night preferences. And recommendations may shift depending on whether you’re browsing on a smartphone, a tablet, or a smart TV.
By analyzing contextual signals, AI can create special and surprising moments of serendipity that feel intuitive and timely.
The Business Case for Serendipity
For businesses, AI-powered serendipity isn’t just a feel-good feature—it’s a game changer. Companies that successfully implement serendipitous recommendations can expect rise in user engagement. Users are more likely to interact with platforms that consistently surprise and delight them. Netflix, for example, attributes a significant portion of its user retention to its sophisticated recommendation algorithms.
In e-commerce, introducing unexpected yet relevant products often lead to impulse purchases. Amazon’s “Customers who bought this also bought” feature generates billions in additional revenue annually.
Serendipitous experiences also create emotional connections with users, fostering long-term brand loyalty. A user who feels understood by a platform is less likely to switch to competitors.
As personalization becomes standard, serendipity offers a way to stand out in competitive markets. Platforms that can consistently surprise users will dominate the market that is driven by attention economy.
Industries Leading the Charge
Entertainment and Media – Video streaming platforms like Spotify, Netflix, and YouTube are pioneers in the space of AI-powered serendipity. By analyzing user behavior across text, audio, and visual inputs, these platforms create hyper-personalized discovery experiences that keep users engaged for hours.
E-Commerce – Online retailers like Amazon and Shopify are leveraging AI to predict not only what users want to buy but also what they didn’t even know they needed. Multimodal systems analyze purchase history, browsing behavior, and product imagery, to surface surprising yet relevant suggestions.
Travel and Hospitality – AI-powered serendipity is transforming how users discover travel destinations, hotels, and experiences. Platforms like Airbnb have leveraged machine learning to recommend stays or activities based on user preferences, visual cues, and even act on seasonal trends.
Social Media – Social platforms are embracing serendipity to enhance user feeds. TikTok, for example, relies on AI to surface content that feels uncannily relevant—even if it’s generated from the creators the users do not follow.
Challenges and Ethical Considerations
While AI-powered serendipity offers immense potential, it also raises important questions. The more data AI collects to create serendipitous experiences, the greater the risk of privacy breaches without a question, especially taken without consent. Transparent data practices are paramount to maintain user trust.
Second is algorithmic bias. AI systems must be carefully trained to avoid reinforcing prejudiced stereotypes or excluding certain user groups based on social or cultural parameters.
Lastly, there are risks of over-personalization. Overly aggressive recommendations can make users feel invasive, eroding the user experience and trust.
The Future of AI-Powered Serendipity
As AI continues to evolve, the potential for serendipitous experiences will only grow further. Emerging technologies like multimodal transformers, advanced agentic paradigms and self-supervised learning promise even more sophisticated models capable of understanding the full spectrum of human choices.
Soon, we might see AI systems that can successfully, beyond experimentation, generate recommendations in augmented and virtual reality environments, creating immersive, serendipitous experiences; use emotion recognition to adapt suggestions based on your mood in real time; and integrate ethical personalization frameworks into the AI design process to ensure fairness and transparency in recommendations.
Ultimately, AI-powered serendipity is about more than just technology. It’s about creating moments of delight, discovery, and connection in an increasingly digital world. For businesses, it’s an opportunity to not only meet user expectations but exceed them in ways that feel almost fantastic and magical.
The question isn’t whether serendipity will shape the future of AI—it’s how businesses will leverage it to surprise us next.