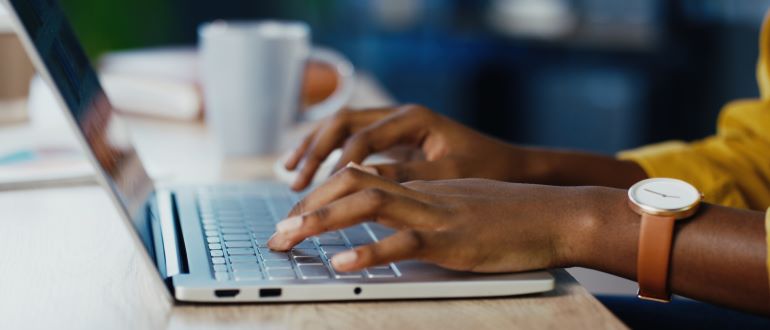
Integrating artificial intelligence (AI), machine learning (ML) and cloud computing is not just a technical feat; it’s a gateway to unprecedented productivity, scalability and creativity. This transformative link between AI, ML and cloud computing holds immense potential for data warehousing and corporate intelligence professionals. To take advantage of all integration can offer, it is essential to understand the drivers behind AI/ML integration, address the challenges faced and highlight the exciting future trends that will redefine the industry.
The Evolution of Cloud Computing
Cloud computing has drastically progressed over the past decade, transitioning from a novel concept to an essential component of modern IT architecture. Technological advancements and the increasing role of AI and ML in optimizing cloud operations contribute to this shift. The need to support AI and ML workloads drove the adoption of resilient, flexible hybrid and multi-cloud systems. It also led to the use of serverless architectures and edge computing—changes that promise to cut latency and costs.
COVID-19 accelerated cloud computing adoption, enabling businesses to adjust to remote work environments while ensuring operational continuity. As highlighted by legislative frameworks like the General Data Protection Regulation (GDPR) in the European Union or the Health Insurance Portability and Accountability Act of 1996 (HIPAA) in the United States, the necessity for data security and compliance has further influenced cloud strategies.
Drivers of AI/ML Integration With Cloud Computing
Multiple factors have driven AL and ML integration with cloud computing, including:
- Hardware advancements. Thanks to the emergence of strong, reasonably priced graphics processing units (GPUs), complicated AI and ML models can now be processed. Cloud companies open advanced computing capabilities to a broader range of users by including these hardware capabilities in their services.
- Open-source frameworks. Tools like TensorFlow or PyTorch have democratized AI and ML for developers who may not be AI experts but want to quickly build models with deployment capabilities. These frameworks and cloud resources make fast experimentation and development possible.
- Data accessibility. The exponential data growth generated by numerous sources provides ample material for training AI and ML models. Abundant data and efficient algorithms are driving the adoption of AI/ML across industries.
- Business needs. The demand for automation, improved decision-making processes, and customer satisfaction enhancement necessitate incorporating AI and ML into numerous company activities (Figure 1). Adobe claims that AI-enabled personalization can boost customer satisfaction by 30 percent.
- Cost savings. A Deloitte survey found that 83 percent of AI-using companies achieved substantial or moderate economic benefits, with 66 percent reporting cost reductions (Figure 2).
Figure 1: AI implementation enhances end-user satisfaction.
Figure 2: Financial impact of AI adoption
Challenges With AI and ML Cloud Implementation
AI and ML cloud implementation only occurs when common challenges are overcome. Limitations in accuracy and the necessity for ongoing improvement might result in costly deployments. Realistic expectations will help organizations, as will sufficient funding for model enhancement. Careful consideration regarding data privacy, ethical issues and deliberations about using private data for model training is also essential.
Retaining customer confidence requires strong security measures and transparent data handling procedures. Accordingly, it is important for organizations to establish explicit rules and monitoring methods to guarantee responsible AI and ML implementation.
Some companies face a challenge in implementing AI and ML due to the scarcity of qualified data scientists. In AI systems, bias identification and reduction require specific knowledge and continuous observation. Companies can address these problems by investing in skill development and forming alliances with seasoned third-party AI and ML service providers.
Real-world applications and metrics
AI and ML integration with cloud computing has produced a plethora of successful applications in several sectors (Figure 3):
- Entertainment and media. AI investments for media and advertising are expected to increase to $7.48 billion by 2025 from $2.91 billion in 2020. A well-known entertainment example of an AI application is Netflix’s ability to provide customized recommendations, improving customer experience and satisfaction. Another is Magellan TV’s utilization of AWS MAP and AWS-native AI to internationalize its content library of streaming documentaries.
- Predictive maintenance driven by AI optimizes operations while reducing downtime. Siemens uses AI, for example, to track equipment health and foresee problems before they happen.
- Finance and retail. AI applications in finance and retail include fraud detection, inventory control and personalized marketing, which drive revenue growth. The State of AI in Retail and CPG report from NVIDIA states that AI-powered personalization may boost retail companies’ sales by as much as 69 percent.
- Rapid analysis of enormous volumes of recorded data using cloud-based AI algorithms facilitates medical research, disease management, and rapid diagnostic accuracy.
Figure 3: AI adoption rates by industry
Upcoming Developments and Trends
Future possibilities will be enormous as AI, ML and cloud computing continue to combine (Figure 4). Introducing 5G and 6G networks is predicted to make ultra-low latency and real-time edge processing possible, opening new opportunities for AI-driven automation and analysis showcasing advancements in wireless communication technology (Figure 5).
Technologies in autonomous systems will change industries like hazardous environment operations and space exploration. Quantum computing and AI-as-a-Service (AIaaS) are about to transform how scalable and accessible AI and ML are in the cloud. The urgency for companies to stay competitive is apparent: They have a responsibility to learn to integrate AI and ML into their business procedures and operations.
Figure 4: Projected growth of the AI customer service market
Figure 5: This diagram illustrates the distribution of network latency for various wireless technologies, including 2G, 3G, 4G, 5G and 6G. The plot highlights the median latency (middle line), the interquartile range (box) and potential outliers (dots).
Combining AI and ML with cloud computing is a game-changing advance for companies in many industries. This integration offers theoretical advantages and tangible benefits that can elevate organizations to previously unheard-of productivity levels, scalability and creativity. It is a truly inspiring field of endeavor that is well worth exploring.