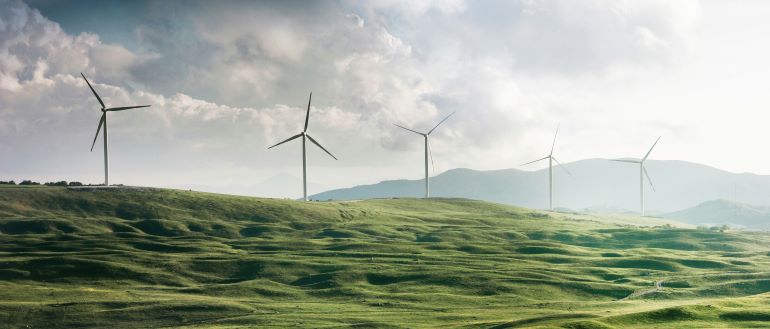
The rapid advancement of artificial intelligence (AI) is often heralded as the next great revolution in tech, promising to enhance productivity, reshape industries and unlock human potential. But an inconvenient truth lies beneath the surface: AI is an insatiable energy glutton, and its hunger is only growing.
As AI models become more sophisticated, their demand for compute resources — and thus power — have skyrocketed. A large AI model, at the training stage, can guzzle as much electricity as thousands of households. To accommodate the demand spike, data centers that power these models are rapidly expanding, consuming vast amounts of energy to power and cool the hardware.
The Energy Cost of AI
AI is not just another software revolution—it is a hardware revolution. Unlike traditional software applications that run on general-purpose processors, AI workloads require specialized hardware such as GPUs and TPUs, which are designed to handle massive parallel computations necessary for machine learning. These chips are incredibly power-hungry and as AI models grow in complexity, the number of these chips required increases exponentially.
Consider the case of OpenAI’s GPT-4, one of the most advanced language models in existence. Training such a model requires thousands of high-performance GPUs running continuously for weeks or even months. The energy consumption of this process is staggering, often measured in megawatt-hours. And this is just for training. Once deployed, these models continue to consume energy as they process queries, generate responses and refine their outputs.
The problem is compounded by the fact that AI is not confined to a single industry. From healthcare to finance, autonomous vehicles to entertainment, AI is being integrated into every modern sector of business. Each new application adds to the overall energy burden, creating a feedback loop where more AI means more energy consumption, which in turn demands more infrastructure, more cooling and more power.
Data Centers: The Silent Energy Giants
At the heart of the AI energy crisis is the data center footprint required to train and refine models. These sprawling facilities house rows of servers used to process and store the data required to build AI models.
Data centers are already among the largest consumers of electricity in the world, and their appetite is only increasing. A single high-density data center rack can consume upwards of 120 kilowatts of power, and some projections suggest that future racks could require as much as 400 kilowatts. To put this into perspective, a typical U.S. household consumes about 10,000 kilowatt-hours per year. By contrast, a single AI data center can easily consume as much electricity as a small city.
The challenge is not just to supply this power but also to vent the heat it generates. Servers running AI workloads consume enormous amounts of power and produce an equal amount of heat. This requires sophisticated cooling systems that themselves require additional energy. Most modern servers use liquid cooling technology but this just moves heat off the chips more efficiently; the data center itself still produces the same amount of heat. New ideas like geothermal cooling or reuse of data center heat are beginning to take hold, but these solutions are not yet widespread. In many cases, the heat is simply dumped into the atmosphere, contributing to urban heat islands.
The Renewable Energy Challenge
Proponents of AI often argue that renewable energy can offset its growing power demands, and there is some truth in this. Solar and wind energy are becoming more efficient and cost-effective and are already far cheaper than conventional fossil fuels. But renewables are not a panacea: The intermittent nature of most renewable energy sources means that batteries or other storage media are required. And data centers still rely heavily on traditional power sources, including coal, natural gas, and nuclear energy as a back-stop.
Even if AI data centers transition to 100% renewable energy, the investment will be massive. Solar panels and wind farms require vast amounts of land, and battery storage technology is only advanced enough to provide consistent power at data center scale. It is not yet clear whether it will be able to support the demands of large-scale AI model training. Even if companies invest in renewable energy, the sheer pace of AI expansion means that energy consumption will continue to outstrip supply.
The Nuclear Option
Given the limitations of renewables, some tech giants are turning to nuclear power as a potential solution. Small modular reactors (SMRs) are being touted as a way to provide high-density power to AI data centers, but this technology is even less mature than batteries. Indeed, no SMR has yet been deployed in production, and most of the hyped advances are still confined to the lab.
At this point, we are still talking about conventional large-scale fission reactors. Microsoft, Amazon, Google and others have expressed interest in using conventional nuclear power plants, but these have many drawbacks. While nuclear power is a low-carbon energy source, regulatory hurdles, public skepticism and the long lead times required to build new reactors make it difficult to deploy. And the electricity from conventional nuclear power stations is much more expensive than fossil fuel or renewable alternatives. Even if nuclear becomes a viable option for AI, it would take decades before it could meaningfully impact AI’s energy consumption.
The Future: Efficiency or Excess?
The best way to mitigate AI’s energy crisis is through efficiency improvements. Advances in semiconductor technology have led to more energy-efficient chips, and new AI architectures could be designed to require less computational power. However, history suggests that efficiency gains are often offset by increased demand, a phenomenon known as Jevons’ Paradox. As AI becomes more efficient, it will become more accessible, leading to even greater adoption and, paradoxically, even higher energy consumption.
Another approach is to rethink how AI is deployed. Instead of centralizing AI workloads in massive data centers, companies could explore edge computing, where AI processing is distributed across smaller, localized devices. This could reduce the need for energy-intensive data transfers and alleviate some of the strain on centralized power infrastructure.
The Inevitable Reckoning
The reality is that AI’s energy consumption is a societal challenge, not just a technical one. As the use of AI continues to grow, we must ask ourselves how much energy are we willing to devote to it. Are we prepared to build more power plants, expand our electrical grids and accept the environmental trade-offs that come with AI’s insatiable hunger? For now, the trajectory is clear: AI will continue to drive massive energy consumption, and our current solutions are not keeping pace. We must pursue renewable energy, nuclear power and radical efficiency improvements to balance its potential with the energy costs. Otherwise, we risk creating a future where the benefits of AI are overshadowed by the very real consequences of its power demands.