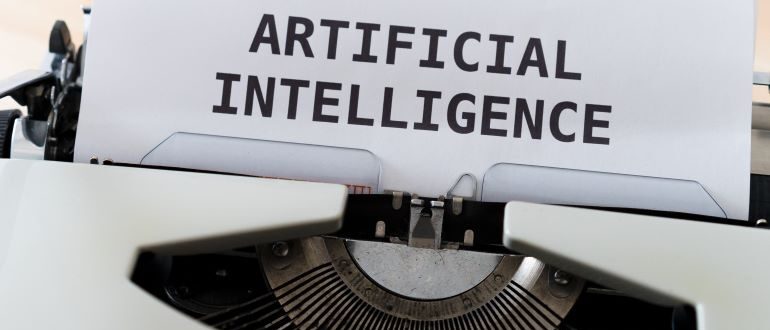
Since the release of Open AI’s Chat GPT in November 2022, leaders have been confronted with a pressing dilemma: How do they seamlessly integrate these tools into their organizational processes, prove to stakeholders that the business is staying current with emerging trends and that they’re adapting their business to technological advancements? Before jumping on the AI hype train, leaders must understand that AI implementation done too quickly, without recognition of its capabilities and drawbacks, can lead to far-from-desired outcomes. But, with the proper care and responsibility, AI can increase productivity and efficiency for organizations willing to jump on the trend.
Quick Implementation Isn’t Always Good
Even before Chat GPT came about, Microsoft had one of the most famous cases of AI gone wrong that should have been a warning to all who seek to implement this technology without foresight. In March of 2016, Microsoft released its AI chatbot, Tay, which used Twitter interaction as training data for its machine learning algorithms. Tay was to assume the persona of a teenage girl as an experiment in conversational understanding. Within the first 24 hours, users began tweeting racist, misogynistic and antisemitic comments to Tay, which the chatbot incorporated into its material. Following this blunder, Microsoft admitted, “Although we had prepared for many types of abuses of the system, we had made a critical oversight for this specific attack. As a result, Tay tweeted wildly inappropriate and reprehensible words and images.”
Because of Microsoft’s large footprint in the technology industry, this incident didn’t wipe them off the map, but rather tarnished their reputation. On the other hand, if a smaller technology company was responsible for this error, it could have meant the end of their business and reputation entirely.
As ChatGPT and generative AI integrations become popular, it’s imperative for leaders to carefully assess the utility and accountability of incorporating ChatGPT and generative AI technology into their offerings. IT teams need to concentrate on problem areas and establish clear-cut guidelines to use this technology soundly, instead of blindly embracing the hype. By drawing lessons from others, leaders can ensure they steer clear of the same pitfalls.
Train on High-Quality Data Sets
It’s crucial to recognize the significance of the data used in AI training. Microsoft could have used a more comprehensive, high-quality dataset for Tay, which would have not only prevented the negative consequences of this blunder but also provided valuable insights for future AI integration. Leaders at Microsoft should have understood that data quality is more impactful on the success of AI than, sometimes, the quantity of data. Although it may be challenging to achieve high-quality data at the scale required for AI, it’s essential for AI solutions that are reliable, responsible and useful, especially in the workplace.
When training your own model, building a quality dataset is among the most challenging tasks, and, in fact, is the cause of an extremely high cost of building and training such custom models. When relying on “off-the-shelf” pre-trained large language models, an assumption is being made that the model is trained on a very large body of data (for example, documents publicly available on a large number of websites) and, therefore, quality can be achieved by quantity. While this approach is constantly being tested by an array of consumer-level and corporate-level operations like Open AI’s ChatGPT, Google’s Bard and others, it’s still important to understand the underlying assumption and act accordingly.
Test and Validate Before Wide Use
Testing and validation are crucial due to the novelty of AI technology and the need to further develop its capabilities. Additionally, high-security fields such as cybersecurity and healthcare require more stringent testing and validation due to the sensitive nature of their work.
If models aren’t tested appropriately in the context of cybersecurity, critical threats could be overlooked and lead to cyberattacks, often resulting in the loss of business-critical data and even perhaps shutting down the business entirely. In healthcare, AI hasn’t taken over for physicians, but it has played an active role in diagnosis. AI models have been trained to read test results and scans to flag potentially dangerous cases for further examination. However, if these models aren’t tested and validated properly, those results and scans could be overlooked, potentially leading to late diagnosis for a life-threatening condition. Although many leaders want to be the first to punch in their respective industries in utilizing AI, overlooking this crucial step can hurt their business more than it could help it.
“What good is the use of AI if we have to validate every step it makes?” some may ask. The reality is that not every step requires a validation, but a smart risk-based approach should be taken to stream the reliance on AI in some decision junctions, whereas making the validation mandatory in others. Just like with any risk-based model, things are not binary.
Monitor and Maintain on an Ongoing Basis
As business needs shift, so must the technology used for it. It’s not easy to develop and utilize an AI model, so ensure it can be useful and effective long-term by prioritizing monitoring and maintenance. By monitoring AI models on an ongoing basis, leaders can rest assured knowing that their business is running smoothly and according to plan. Furthermore, monitoring these systems constantly offers an opportunity to improve the model through maintenance, making it more effective.
Don’t Avoid AI– Just Be Smart About it
While it’s clear that implementing AI can go wrong without proper planning and strategy, avoiding AI at all costs is not the answer. Similarly to when the internet or the cloud were introduced, many avoided fully leaning into these new technologies, but as we know now, these areas are standard in businesses across industries. This shift in thinking is likely to happen with AI, too.
This technology is only gaining in popularity as stakeholders increasingly ask leaders to create plans for AI implementation or learn more about how AI can help them reach their business goals– it’s almost unavoidable. So, leaders should not be weary of this technology entirely, but rather, take others’ mistakes as learning opportunities. Eventually the use of AI will be second nature, and it should be the goal of every professional to understand its utility, specific to their needs.