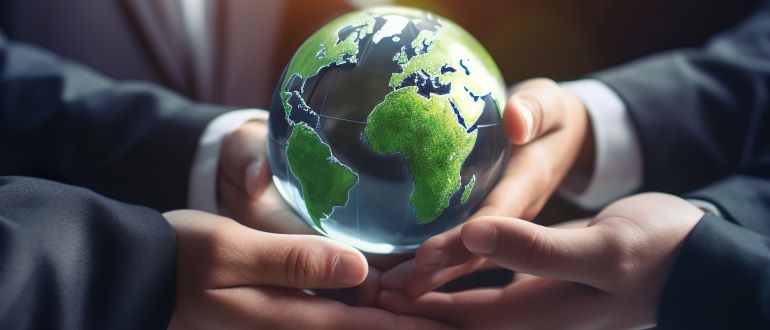
Artificial intelligence (AI), automation and machine learning all promise to redefine business operations in complex sectors—and it doesn’t get more complex than operations for the energy, utilities and resources (EUR) sector. Current predictions foresee that energy demand will double by 2030—projections that not only our current energy grid cannot handle, but that only 25% of this increased demand can be solved by renewable energy. Even with a three-fold growth in renewable energy supply, a 42% shortfall is predicted by 2050.
In the coming years, the sector has a number of pressing industry challenges to contend with—increased energy demand, a need for integrated systems with embedded AI and automation, the rise of sensor and smart meter deployments, and the growing requirement for carbon capture and storage.
AI, ML and automation hold great potential in transforming a traditional sector into a sustainable and efficient industry—from data collection on scale, to analysis for aging infrastructure and essential ESG reporting.
Don’t underestimate the link between data and sustainability.
In the EUR sector, data is key. When collated, analyzed and then utilized, companies can reap the benefits of improved decision-making, enhanced customer personalization and the simultaneous need to become more efficient, streamlined, environmentally conscious and ethical.
When addressing the twin challenges of energy and sustainability at scale, AI and automation can draw on vast amounts of data—far more than humans could reasonably process—and analyze this data to reveal patterns of energy use and areas of inefficiency. AI can help companies incorporate renewable power sources, manage better decisions about EV charging infrastructure, and reach their sustainability objectives, all while cutting energy costs.
Automation Needs a Purpose
But simply deploying AI and automation without a clear roadmap will only get the sector so far. The intrinsic link between data and sustainability requires a shift: Mindset and culture first, then come solution suites. Automation through AI has been sold as an efficiency and sustainability-inducing silver bullet, but without a clear mission in place, EUR companies will find themselves on a digital transformation journey to nowhere—it’s automation without a mission.
Solutions such as machine learning, feed off the questions being asked of it, and the data being presented as a result. Without the initial culture geared up to not only ask the right questions, but push towards achievable and clear goals on the sustainability front, the tech simply won’t be able to perform to its highest standard.
Dig for data with purpose, optimizing assets to improve sustainability.
A common trend across all industries has been the collection of masses of data, with no intended purpose thereafter. Businesses have rushed to collect more data, without even a structure in place to enable the sector to put it to good use—which actually works against the concept of sustainability.
For example, companies spend extra time and money to keep up with their own data deluge as it comes out the other side of the automation filter—be it a new data scientist hire, or additional technology to try and better connect functions. It derives from a misunderstanding of the tech that has been originally deployed to theoretically do all of this hard work for them—namely, AI.
When it comes to optimizing existing EUR assets, it’s what companies do with this data that will optimize sustainability efforts. The electrification from renewables provides a sustainable energy solution, but it also poses challenges around resilience. While renewables provide an opportunity to update and build the grid back better, the short-term reality will see the lifetime of existing ageing assets needing to extend further, demanding robust maintenance and monitoring to remain reliable and safe.
AI Holds the Key to Asset Lifetime Optimization
The key to asset lifetime optimization lives within the data—collected via sensors, scanners, or customer demand reports. When data is coupled with AI based predictive analytics, organizations can make confident investment decisions on the most critical areas of the business. AI-enabled data analytics capabilities must work dynamically and autonomously to pull data and enable users to leverage insights at the right time for continuous improvement. While ensuring the health of assets, AI-enabled data analytics capabilities also correlate historic operating temperature, pressure and maintenance data with outages, revealing the most uptime-critical assets and helping to plan appropriate condition-based maintenance.
There’s nowhere to hide in the ESG race; transparency is vital.
The need to not only become more efficient, but to clearly and outwardly display how the company is financially, socially and environmentally sustainable is a growing market differentiator. Customers seek transparency in the products and services they are purchasing, and demand to know more about how their service providers are being run.
The same levels of transparency apply to other stakeholders within the business. From a reporting perspective, regulators are likely to request more audits and reviews for sustainability reasons—this is where AI-driven data collection and analysis will be key in producing these records. Ultimately, sustainability at the back end needs to be visible, transparent and auditable, which of course, can only be achieved if the initial goals are equally clear and laid out from the beginning.
There’s nowhere to hide in the ESG race and the reality is that there is no coincidence or foregone conclusion when it comes to the upshots of automation tools.
Building a Sustainable EUR Roadmap for the Future
The EUR industry is facing a worsening reality of climate issues, and organizations must make drastic changes to increase their sustainability and prioritize renewable energy.
AI and automation are set to revolutionize data collection and analysis in the quest to improve ESG reporting and sustainability progress tracking. However, this isn’t a one-stop-shop, the EUR sector needs to implement permanent changes to make a real difference.