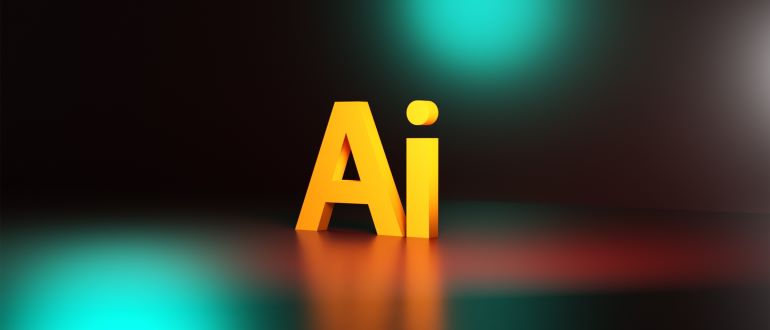
AI represents a massive, generational wave of technology. Yes, there are reasons to be skeptical of any technology—AI included—and yes, it’s important to approach any new technology carefully. But make no mistake: AI is already changing the way companies do business, and both the advantages and risks that come with it are becoming increasingly clear. AI is still only beginning to emerge as a dominant force, which means we have yet to see whether the AI wave will be as big as the birth of personal computers in the 80s, the internet in the 90s, or the mobile era of the 00s. It could be as big as any of them. It could well become as big as all of these waves combined.
With that in mind, enterprises have to actively plan around implementation and usage. AI presents abundant opportunities today – improving customer support and employee productivity, turbocharging marketing efforts, accelerating product development and sales efficiency, automating elements of legal and RFP reviews – with new capabilities emerging on an almost daily basis. At the same time, establishing appropriate “rules of the road” and putting effective AI governance practices in place is essential to the long-term success of any company utilizing AI.
Establishing AI Governance Across the Business
As exciting as the technology is, there are concerns that come along with AI—particularly security, privacy, and data risk-related concerns. There are still plenty of legal and ethical questions that need answering. How are these AI solutions being trained? Where does the data come from? Do biases exist, and can they be accounted for? How is the data they generate being used? Where is it being stored, and how is it being secured? And who ultimately bears responsibility for decisions made by an AI-based system? Failure to answer these questions effectively can result in damage to the brand reputation or worse—regulatory challenges and fines. Organizations need to examine how—and why—they plan to use AI and understand the potential risks that come along with it. That means having a playbook plan for AI governance.
Navigating AI governance requires a thoughtful approach that carefully considers the needs of the organization. New AI capabilities are emerging at an accelerating rate, and business leaders are under immense pressure from both customers and board members to adopt and implement AI quickly. In practice, this creates two distinct categories depending on the risk appetite of the organization.
On the one hand, companies in highly regulated industries (or where there is strong risk aversion in ET) have a tendency to limit AI usage to avoid violating regulations and policies. On the other hand, there are companies taking a Wild West, “anything goes” style approach, effectively allowing any department (or individual employee) to adopt and use any AI-based solutions they want. Of course, in real life, it’s rarely so black and white – most organizations fall somewhere in the middle. They want to lock things down, but a robust “shadow AI” element occurs throughout the organization with little oversight. These organizations are desperate for a better way to manage their AI implementations. Fortunately, effectively governing AI is no longer as difficult as it once was.
A 3-Step Starter Program: Leverage Existing Data Classifications, Inventory Proposed AI Use Cases, and Implement Low Risk, High Reward efforts
Data leakage is one of the biggest risks when it comes to AI usage. A top risk to mitigate and govern in the usage of AI is data leakage – if a public LLM is granted access to confidential or sensitive data, that data might wind up in its training model and be subsequently exposed to competitors (or worse, hackers). Sensitive information like source code, contracts, and other business-critical data should be kept controlled – and far away from AI services (especially those with unclear terms and conditions). Most risk-aware companies have fairly robust data classification policies, with processes in place to determine the sensitivity level of certain data and establish appropriate protections. This is a good place to start for businesses looking to dip their toes into the AI waters: make sure your current data classification program and processes are prepared to account for AI.
With an effective data classification program in place, it’s time to inventory AI use cases within the organization. From there, it is relatively simple to map AI use cases to the data they utilize – from low-impact data to high-impact. The goal here is to identify use cases that have a high level of impact but use low-sensitivity data. For example, businesses are finding that they can leverage OpenAI bots to power meaningful improvements to their security capabilities without using any sensitive internal data. These bots can help make security testing and tabletop programs more dynamic, which strengthens the security team’s ability to recognize, triage, and remediate incidents. Because there is no risk of data leakage, there is very little downside to this use case, making it the perfect example of “high impact, low risk.”
The most challenging part of the process is understanding how to approach AI use cases where the licensing and usage terms of the third party providing the AI capabilities is unclear. It’s important to think carefully about whether capabilities that fall into this bucket need to be used at all, and, if so, how to establish the necessary controls. AI is a fast-moving space, so the ability to roll with the punches is key. An organization using a work management solution with AI capabilities might find that the data ownership rights in its terms and conditions are currently ambiguous. If that solution might touch sensitive data, the organization needs to be able to decide whether to stop using the tool or build the security controls needed to limit risk should those data ownership rights become unfavorable in the future. Trying to forecast what the future may hold is difficult, but planning ahead can help mitigate the potential damage caused by negative outcomes.
AI Governance Ownership and Buy-in
As pressure mounts on today’s enterprises to invest in AI capabilities, it is increasingly important for organizations to understand the risks associated with AI—and the impact they can have. It is essential to have a robust data classification program in place that can be easily linked to AI use cases throughout the organization, helping GRC, Privacy and newly established AI Visionary teams more effectively visualize the policies and controls in place. It is critical to establish transparency throughout the organization, with full understanding around how AI is being used and why certain limitations and controls are necessary.
Finally, and this is the key, it is important to acknowledge that this industry will change, and change rapidly. AI development and implementation has barely gotten started, and it is impossible to predict how the space will evolve in the coming months, quarters and years. But we know for certain that it will change quickly – which means organizations need to prioritize being light on their feet, seeking partners and solutions capable of helping them keep up with the pace of technology amid this dynamic and exciting time of transition.