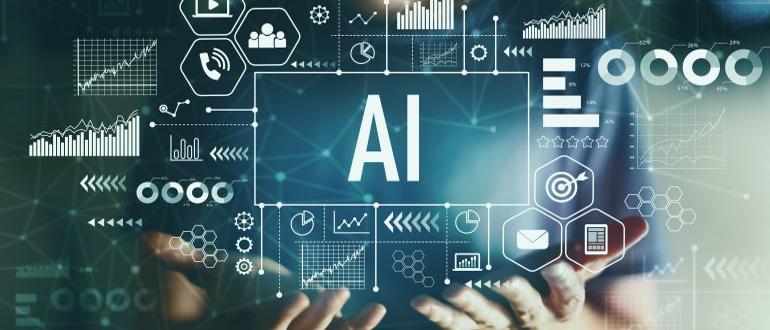
Nutanix today extended its reach into the realm of IT infrastructure for artificial intelligence (AI) applications by adding support for the NVIDIA Inference Microservices (NIM) and NVIDIA NeMo frameworks.
Announced at the .NEXT conference hosted by Nutanix, the frameworks make it simpler to deploy AI inference engines and customize large language models (LLMs), respectively.
These additions to the Nutanix Enterprise AI (NAI) platform extends the capabilities of an integrated platform that they would otherwise have to integrate and maintain themselves, says Lee Caswell, senior vice president for product and solutions marketing at Nutanix.
NAI leverages NVIDIA AI Blueprints, a pre-defined set of customizable workflows, to provide a foundation for building and deploying AI applications. In addition, NAI enables function calling for the configuration and consumption of external data sources to help agentic AI applications, for example, access additional data sources that allows them to deliver more accurate and detailed results. It is based on a repository for invoking multiple LLMs, the NVIDIA AI Data Platform reference architecture, and Nutanix Unified Storage and the Nutanix Database Service offerings that have been integrated into NVIDIA GPUDirect Storage.
Those capabilities collectively make it simpler to reprocess data in a way that allows LLMs to continuously fine tune output, says Caswell. “These are iterative processes,” he says.
NVIDIA NeMo then makes it possible to apply guardrails to how that data is accessed. AI models can filter initial user queries and LLM responses to prevent biased or harmful outputs that might be generated, should the security of a model be compromised.
Overall, the partnership should make it simpler for IT teams to operationalize AI, says Guy Currier, an industry analyst with The Futurum Group. “It is based on what has become a de facto industry standard for AI development, deployment, and operations,” he says.
Recent research from the Futurum Group makes it evident that organizations are struggling with AI infrastructure issues. A full 60% of do-it-yourself (DIY) initiatives fail to scale past pilot stages due primarily to a lack of return on investment (ROI) clarity, according to the Futurum Research survey. Three quarters (75%) of DIY AI projects also have experienced prolonged development cycles and 78% of CIOs cite security, compliance and data control as primary scaling obstacles for AI agents, the survey finds.
Precisely which teams are managing AI infrastructure tends to vary widely from one organization to another. In some cases, a dedicated AI team includes specialists to manage infrastructure. Many of those teams have already built some type of custom IT environment to build and deploy AI applications. In other cases, IT teams are now starting to assume responsibility for managing AI infrastructure on behalf of multiple data science and application development teams.
Regardless of the approach, the amount of IT infrastructure required to run AI applications at scale represents a major investment. The challenge now is determining which AI projects are going to best justify the return on investment in that AI infrastructure at a time when many organizations are much more sensitive to the total cost of IT.