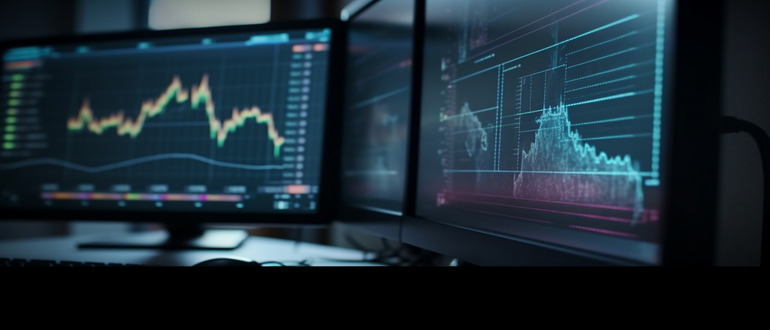
While companies are going to extraordinary lengths to obtain valuable compute power to satisfy the growing appetite of artificial intelligence (AI), a group of vendors have found a way to dip into the existing infrastructure for extra shot of compute.
In a very shop-your-own-closet style, MemVerge has developed a new offering that finds and harvests idle resources trapped within existing GPU stacks, relieving companies of the need to constantly pour money into infrastructure expansion. MemVerge named it Memory Machine AI or MMAI, a cloud-agnostic software solution that allows AI workloads to surf and share GPU resources between themselves.
But MemVerge is not the only company to come up with this workaround. A growing body of vendors are now offering what is gaining popularity as GPU-as-a-service (GPUaaS) that provides enterprises technology to tap into underutilized processing capacity within their current servers.
A global survey conducted by Big Data Wire finds that where 96% respondents plan to build out their AI compute infra, they are hindered by cost and availability challenges with compute wastage and idle costs being their top concerns. The respondents also said that they are unhappy with the job scheduling and orchestration tools they currently use.
GPUs are exceedingly fast and have incredible efficiency if operating close to capacity, but research shows that these expensive equipment see a shockingly low average usage across all runs. Their average utilization rate, according to a Wandb study, is less than 15%.
“AI is making waves across the world impacting our lives and enterprises as we speak…We are looking to adopt AI in a significant way to improve productivity and competitiveness for the enterprises.” Dr. Charles Fan, co-founder and CEO of MemVerge, said, at the AI Field Day event late January where the company unveiled Memory Machine AI.
“Typically, it is done in two ways,” he explained. “One is by leveraging on the API services such as those provided by OpenAI or Anthropic and so on. The second which is the deployment and fine-tuning of those open-source models within the private environment of the enterprises – whether it’s on the cloud or on-prem data centers – where they can serve the AI queries without going to the public and this has the benefit of protecting the data privacy, and in some cases can also be very cost-saving for enterprises,” he added.
The MemVerge Memory Machine AI packs some key features to overcome GPU underutilization for companies doing AI the second way.
While dedicated GPUs have physical limits, MMAI works by creating a virtual compute pool from where GPU resources can be rationed out and repurposed as required.
MMAI features GPU-sharing algorithms whose job is to constantly spot and eliminate idle resources, turning out more GPU-hours of work. To optimize utilization, idle GPUs are allocated to active projects which reduces downtime for the jobs and keeps the GPUs busy around the clock.
“If you’ve run out of your allocation, you can go and borrow somebody else’s assuming they have the resources available, and cross-bill at the end of the day. That creates what we call a “spot market” type of thing – you can beg, borrow, steal and pay them back,” Steve Scargall, director of product management, explained during the presentation.
With a feature called GPU Surfing that moves user jobs uninterruptedly to the next available hardware when the original hardware becomes unavailable, MMAI ensures continuous operation and maximum resource efficiency, MemVerge says.
At the heart of MMAI is the Memory Machine Checkpoint Engine, a stand-alone checkpointing and recovery technology that performs automated checkpointing and restores to ensure zero loss of progress. The engine works to ensure that more workloads are housed in low-cost instances. According to MemVerge, it’s SpotSurfer feature can save up to 90% compute cost.
Automatic suspension and resumption of jobs protects against bottlenecks like out-of-memory conditions, ensuring high-priority tasks continue to run while low-priority ones are paused temporarily to ensure maximum efficiency.
The intelligent job queuing and scheduling controls also ensure that every job gets the maximum hardware performance and efficiency so that project deadlines and user demands are met fully.
“Typical cloud problem is you never know who’s going to want what and when. There’s going to be bursty parts of the day, and you’re going to have batch jobs at night. You need to deal with streaming, real-time kind of workloads,” Scargall noted.
MMAI’s cloud burst management capability allows on-prem and public cloud infrastructures to be linked for managing demand peaks without overprovisioning.
MMAI’s ideal users are IT infrastructure teams and business users like data scientists, researchers and developers, Scargall said, essentially anyone who “shouldn’t care what a GPU is” or “where it lives”.
“We’re trying to get the enterprises engaged in a productivity boost of all the hardware that they’re buying or about to buy and got in the pipeline. The customers that we’ve spoken to are only literally just getting started and have got maybe one or two servers with a few GPUs just to try it and see what they can do with it. So that’s certainly a target audience to us because…everybody has Kubernetes knowledge, but they don’t really know much about GPUs,” he said.