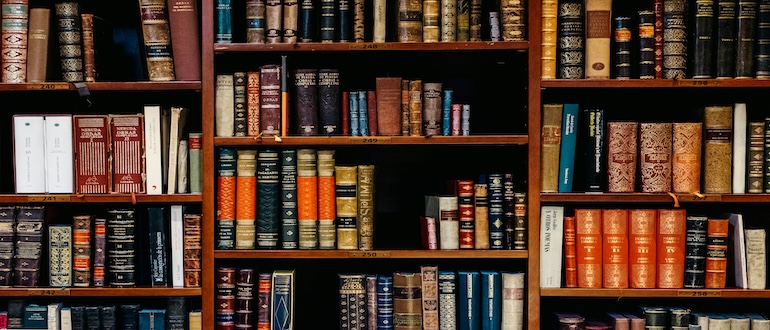
For thousands of years, the historical, cultural and social heritage of Jewish culture has been preserved and promulgated through sacred texts, and now artificial intelligence (AI) is joining the conversation.
“Technology has long played a critical role in bringing Jewish people closer to Jewish texts and tradition, and our AI innovation is the latest leap forward in that journey,” said Daniel Septimus, CEO of Sefaria, a non-profit, online open-source digital library of Jewish texts, offering free content. “By investing in tech innovation, we ensure the vast library of Jewish texts is ready for the AI era.”
By creating a specialized Large Language Model (LLM), the company has preserved the history of the sacred texts of Judaism into “1,000+ curated encyclopedia-style pages that allow any learner anywhere to quickly get answers to modern-day questions, like what Jewish texts have to say about love, gratitude, and grief. Using AI, Sefaria has also written a learning guide for a first-century text and developed an advanced citation linker – both streamlining the learning experience for learners worldwide.”
The process to what Sefaria has created has been in the making for almost 15 years. Co-founders Joshua Foer and Bret Lockspeiser, met back in 1999, when they went on a group trip to Israel, as teens. Mr. Lockspeiser went off to Google, where he became a project manager, and Mr. Foer embarked on a career as a journalist, where he became an author, but they reconnected years later “over a shared frustration about the state of Jewish texts online.”
Google searches about the Torah back then would yield dubious results, many that were antisemitic or without any true connection to Jewish texts, Mr. Lockspeiser said.
The two men went to work digitally preserving the texts. That job seemed daunting, because it involved taking over 3,000 years of history into digital form, but through teamwork, and Mr. Lockspeiser’s experience at Google with massive volumes of information, the Sefaria Library was founded in 2011, with over 100 million words of texts. The Library provided translations, commentary and connected related texts to each other.
“These texts are the source of Jewish peoplehood, of Jewish culture, of Jewish law, of Jewish values,” Mr. Foer said back in 2017. “We’re now living in a digital world and we are the generation that has been charged with sheparding these texts, this ancient tradition, into a new digital era.”
Much has changed since then in the world of technology. Programs were created that can understand, generate and manipulate human language. LLMs were perfect for the sort of projects that the Sefaria Library brought forth.
Through the application of AI to the library, searches can unlock even the most obscure Jewish texts. “Instead of opening a specific book, learners can now search the library by topic and find a collection of all the texts from the library on a single theme . . .”
Searches for topics such as friendship, parenting and food, can yield concise information.
“The Jewish tradition is full of questions,” said Sara Wolkenfeld, chief learning officer at Sefaria. “Our new features provide a wide range of sources that speak to the curiosity of Jewish learners, parsing through centuries of texts to find meaning at the intersection of Jewish text and their own lives.”
Mr. Foer and Mr. Lockspeiser now serve on the Board of Directors for Sefaria, which now has a team of over 40 individuals, including software engineers and translation and research specialists.
A University of Michigan “Technology Assessment Project” from April 2022 describes how LLMs function and how they are tailor-made to tackle massive amounts of data.
“Developing an LLM involves three steps, each of which can dramatically change how the model understands language, and therefore how it will function when it is used. First, developers assemble an enormous dataset, or corpus, of text based documents, often taking advantage of collections of digitized books and user generated content on the internet. Second, the model learns about word relationships from this data. Large models are able to retain complex patterns, such as how sentences, paragraphs, and documents are structured.”
“Finally, developers assess and manually fine-tune the model to address undesirable language patterns it may have learned from the data. After the model is trained, a human can use it by feeding it a sentence or paragraph, to which the model will respond with a sentence or paragraph that it determines is appropriate to follow.”