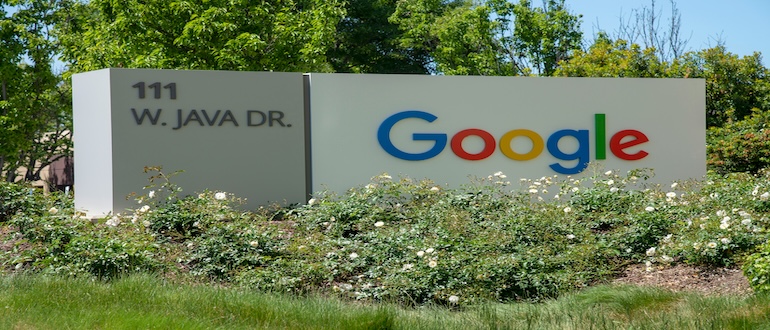
Google today unveiled an interoperability protocol for artificial intelligence (AI) agents, along with a bevy of AI agents that makes use of version 2.5 of the Gemini large language model (LLM) to automate a range of tasks across the company’s entire cloud portfolio.
Announced at the Google Cloud Next ‘25 conference, Google is developing an open Agent2Agent (A2A) protocol to enable AI agent interoperability with support and contributions from more than 50 technology partners namely, Atlassian, Box, Cohere, Intuit, Langchain, MongoDB, PayPal, Salesforce, SAP, ServiceNow, UKG and Workday; and leading service providers including Accenture, BCG, Capgemini, Cognizant, Deloitte, HCLTech, Infosys, KPMG, McKinsey, PwC, TCS and Wipro.
The goal is to facilitate the development of a wide range of AI agents that are trained to specifically automate tasks spanning everything from coding to invoking Big Data analytics applications, says Amin Vahdat, Google’s vice president and general manager of ML systems and cloud AI. “We’re working on this with more than 50 partners,” he says.
At the same time, Google is also making available tools for building and deploying agents in addition to providing more access to AgentSpace, a framework for building AI agents that had been only available to a limited set of ends. That framework can also now be invoked via other Google tools for building applications and managing data.
Google also unveiled a 7th generation Tensor Processing Unit (TPU), dubbed Ironwood, and updated its AI Hypercomputer, to provide five times more peak compute capacity and 6 times more high-bandwidth memory to applications using the Google Vertex AI service.
Ironwood TPUs come in two forms using either 256 chips or 9,216 chips, each available as a single scale-up pod, with the latter pod delivering a staggering 42.5 exaFLOPS of compute, capable of rivaling standalone supercomputers. A new 400G Cloud Interconnect and Cross-Cloud Interconnect offers up to 4 times more bandwidth than an existing 100G cloud network.
A new Cloud Storage zonal bucket also enables IT teams to colocate their primary storage alongside TPUs or GPUs to provide up to 20x faster random-read data loading than a Cloud Storage regional bucket. Additionally, there is a new more consistent read cache, dubbed Anywhere Cache, that works with existing regional buckets to cache data within a selected zone. It enables responsive and real-time inference interactions by keeping data close to accelerators, reducing latency by 70%, according to Google.
Google is also making available Pathways distributed runtime, originally developed by Google DeepMind to run AI inference engines. The distributed runtime is available on Google Cloud.
Finally, Google has updated the Google Kubernetes Engine (GKE) service that many AI inference engines are deployed on to make it easier to manage a fleet of Kubernetes clusters.
Google appears to be making up for some lost AI ground. After arguably inventing the first transformer on which generative AI models are based, the company has been rapidly regaining ground subsequently lost to rivals,” says Nick Patience, vice president and practice lead for The Futurum Group. In fact, Gemini 2.5 now ranks at the top of LLM benchmarks, but organizations still need to be sure their specific use case lends itself best to one LLM versus another, he added. “Benchmarks don’t necessarily reflect real-world use cases,” he says.
There are, of course, no shortage of options when it comes to AI platforms. The challenge now is determining which one to employ based on the total cost that might be incurred.