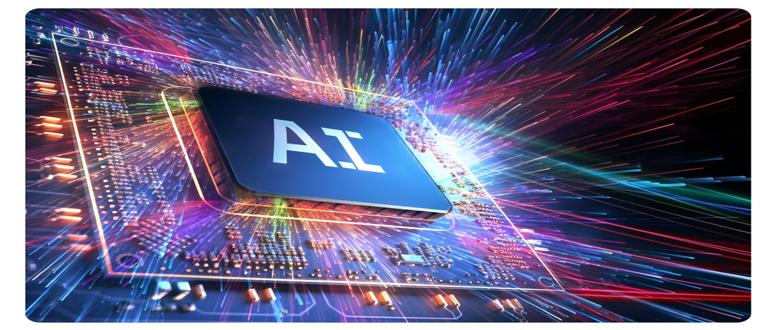
As a society, we are currently in the process of outsourcing thousands of daily tasks to artificial intelligence (AI). Some of these tasks are fairly mundane, such as asking Alexa for restaurant advice. Some, particularly within industries like healthcare and finance, are far more serious. AI has genuinely transformative applications in these highly sensitive areas, but the stakes have slowed adoption.
Part of the problem here is that most AI applications operate on a “black box” model. Even when their outputs are objectively correct, people are troubled by their inability to know how they arrived at a given decision. Without the ability to show reasoning, AI hesitance will remain a feature of the modern business landscape and adoption will continue to be staggered and piecemeal.
In highly regulated industries, the problem goes even deeper. A major hospital system or financial institution cannot blame the machine if something goes wrong due to an AI decision. They are the ones who will have to take responsibility. At a more mundane level, regulated institutions must be able to provide detailed decision-making data for compliance purposes, and here, too, much current AI technology is lacking.
None of these problems is intractable. Transparent and humane AI is possible, and countless researchers are at work on bringing it to life. Taking their ideas seriously is a prerequisite to overcoming wide-scale AI hesitancy and unlocking this technology’s full potential.
The Rise of Explainable Artificial Intelligence (XAI)
In theory, an industry like health insurance is ripe for AI transformation; it deals in standardized forms that are readily comprehended by current LLM-based technology. Indeed, healthcare insurance companies are adopting this technology en masse, with prior authorization determinations increasingly outsourced to AI.
But this rollout has been rocky: According to the American Medical Association, 61% of physicians believe that payers’ use of AI is increasing prior authorization denials. Some estimates suggest that AI tools have been accused of producing rates of care denial sixteen times higher than the average.
This is unfortunate for many reasons, chief among them that AI undoubtedly can play a valuable role in prior authorization assessments. The problem, here boils down to explainability. People don’t know why certain decisions about authorization are being made, which makes those decisions very difficult to trust.
This concern motivates research in the field of explainable artificial intelligence (XAI), which aims to open the black box and grant human users actual insight into the outputs of machine learning algorithms.
Per IBM, XAI consists of three main methods. One is prediction accuracy, which uses simulations to determine the accuracy of the model’s results. Another is traceability, the ability to track and document each stage of the process that leads to an eventual output. Finally, there is the human element, which is known as “decision understanding.” This involves training staff to understand the AI model’s processes and outputs.
Together, these components of XAI can go a long way toward increasing trust in AI applications. They are increasingly baseline requirements for AI decision-making in high-stakes, regulated fields. But they can only get us so far. If we want to quell society-wide fears around this technology, we need to drastically widen the pool of human intelligence.
An Interdisciplinary Approach
We tend to think of building an AI model as a technological challenge, but it is also a human challenge. This technology will affect everyone. Building it with maximum transparency means moving beyond developers and data scientists to bring a wide range of specialists into the process.
Anthropologists, biologists, sociologists and historians each have a role to play in this important process. Each brings a fresh perspective to the question of how technology shapes human behavior, societies and cultures. And each, in turn, can help ensure that burgeoning AI models can effectively and ethically serve the needs of the millions of people whose lives they are soon to impact.
Of course, there is precedent for this. For instance, the moderation councils instituted by the major social platforms last decade stemmed from an acknowledgment that technology is never neutral and that it is prone to the same biases and errors as its human creators. In the case of AI, whose applications touch on every aspect of society, this interdisciplinary approach is even more important.
This is not merely about placating skeptics or ensuring ethical practices, although these are important. By drawing on the domain-specific knowledge of trained experts, those building AI stand to improve the technology itself. The more transparent and humane the model-building process, the likelier its outputs will be accurate. By integrating ethics, empathy and accountability into every stage of the development process, we ensure this technology evolves effectively and responsibly, bridging the AI trust gap and taking us one step closer to a society that works for all.