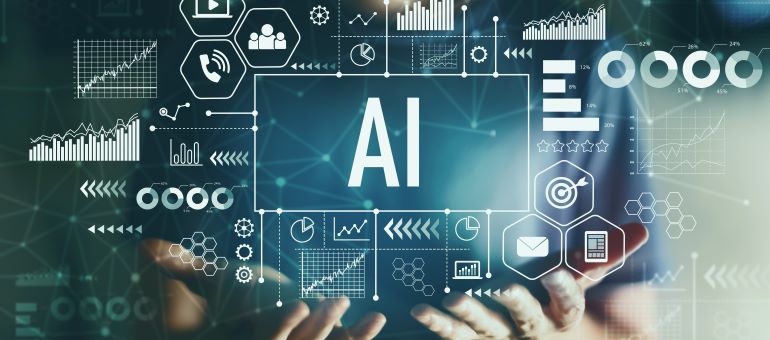
Over $2 billion has been invested in agentic artificial intelligence (AI) startups in the past two years. And as major cloud providers race to develop their own offerings, Deloitte predicts that 25% of the companies using GenAI will launch agentic AI pilots this year—rising to 50% by 2027.
As organizations move beyond early GenAI experimentation to deploying autonomous, multimodal AI agents, the demands on IT infrastructure is intensifying quickly. Instant decision-making, ultra-low latency and immense compute power are the new requirements—and legacy infrastructure simply can’t keep up.
Agentic AI is here to stay, and enterprises that haven’t modernized their infrastructure may find themselves at a disadvantage.
Infrastructure Demands of Agentic AI
Leadership might be ready for the agentic AI revolution, but for many, their infrastructure tells a different story. As enterprises scale their AI operations—deploying hundreds of models into production—the strain becomes increasingly evident, creating bottlenecks instead of breakthroughs.
We’ve already witnessed the infrastructure challenges with GenAI adoption—it’s complex and expensive to scale, and difficult to fine-tune for specialized business needs. In fact, only around one-third of the executives in a Hitchi Vantara survey believe their existing infrastructure is up to snuff. These challenges are magnified with agentic AI.
Its independent reasoning, decision-making and action places even more pressure on enterprise infrastructure, particularly for use cases that require deep, domain-specific knowledge. AI agents must process vast amounts of data with minimal delay, requiring far more compute power than traditional IT systems can support. While large language models (LLMs) serve as the foundation of agentic AI, advanced capabilities like chain-of-thought reasoning and multimodal processing (text, image and speech recognition) increase computational demands.
Real-time inferencing further pushes the limits of CPU and GPU resources, with organizations citing insufficient capacity (65%), data locality challenges (53%) and storage performance issues (50%), according to an S&P Global Market Intelligence study commissioned by 451 Group and Vultr. These limitations can delay critical workloads, increase costs and stall AI maturity.
Gartner forecasts highlight the urgency of modernization: By 2028, 33% of enterprise applications will incorporate agentic AI (up from less than 1% last year), 15% of daily work decisions will be made autonomously by AI, and AI agents will handle one-fifth of all digital storefront interactions.
The Shift to AI-Native Infrastructure
To meet these demands, enterprises are moving beyond rigid, legacy IT systems and adopting flexible, AI-native models designed for high-performance computing. According to Deloitte’s 2025 Technology Industry Outlook, hybrid cloud strategies—blending cloud, on-prem and third-party resources—will be critical for balancing scalability, security and performance as AI adoption accelerates.
Additionally, many are extending operations to the edge where AI training and inference increasingly take place. Edge computing is a game-changer for agentic AI, reducing latency around decision-making. A recent study found that over 80% of AI and IT professionals plan to expand AI edge operations within the next year, gaining a competitive edge over those still relying on traditional, centralized models.
Four Critical Considerations for Scaling Agentic AI
To fully embrace what agentic AI might mean for operations, enterprises need to modernize their infrastructure. A next-generation AI stack, built on a hybrid, edge-first approach, is key.
This modern AI infrastructure includes four key elements:
- Scalable, Real-Time Inference: If agentic AI is the “what” and edge computing is the “where,” serverless inference is the “how.” Serverless inference provides flexible, high-performance computing at the data center edge, allowing AI agents to operate closer to end users without the need for extensive infrastructure management. Unlike traditional cloud-based models, serverless inference dynamically optimizes and auto-scales AI workloads, ensuring fast, real-time decision-making while controlling costs. By eliminating the need for dedicated infrastructure, organizations can deploy AI models seamlessly without worrying about underutilized resources.
- Silicon Diversity for Cost Efficiency: AI agents must operate in real-time, which requires optimized CPU, GPU and AI chip resources distributed across cloud regions. However, a one-size-fits-all compute approach is inefficient. Enterprises must adopt silicon diversity, selecting the most cost-effective hardware for each AI lifecycle stage. For example, inference workloads may run best on AI-optimized accelerators, while model training benefits from specialized GPUs. The ability to compose infrastructure with different types of AI chips on demand allows enterprises to balance cost, performance and efficiency. Cloud providers and hardware vendors increasingly offer solutions that allow enterprises to take advantage of this diverse silicon landscape.
- Real-Time Data Streaming and Integration: Data is the lifeblood of autonomous decision-making. AI agents require instant access to relevant data, making low-latency architectures essential. Real-time data streaming, cloud-native AI pipeline and feature stores enable AI agents to collaborate and make rapid decisions. A data mesh approach is often well suited for these architectures, as it decentralizes data ownership and enhances scalability. Since agentic AI is inherently event-driven, adopting event-streaming platforms like Kafka and Pulsar ensures that agents can react instantaneously to environmental changes. As data volumes grow, robust governance becomes essential to ensure compliance and privacy while maintaining app performance—making local data access a critical requirement for both AI agents and regulatory standards. Enterprises must implement data lineage tracking to ensure traceability, data quality monitoring to maintain accuracy, and access controls to secure sensitive information. Without these measures, AI deployments can quickly become unmanageable and non-compliant with regulatory standards.
- Vector Datastores and Retrieval-Augmented Generation (RAG): AI agents need access to a wide range of data sources, including unstructured vector databases and structured relational databases. Retrieval-augmented generation and vector databases are crucial for ensuring AI agents operate with up-to-date and relevant information. Agentic AI thrives on proprietary knowledge, and businesses must leverage vector databases with RAG to harness their unique datasets while maintaining data security. RAG enhances AI models by allowing them to pull in domain-specific, current information, ensuring responses remain relevant and accurate. Expanding RAG capabilities to multimodal data sources (e.g., images, audio, video) will further improve AI agent capabilities, enabling them to process and act on a broader spectrum of information.
Building Today for Tomorrow’s Success
Agentic AI has enormous potential to transform business operations, driving productivity, enhancing customer experiences and improving profitability. However, without the right architectural foundation, scaling agentic AI quickly becomes unsustainable—echoing the challenges seen with GenAI adoption. A modern AI stack—one that incorporates serverless inference, silicon diversity, data streaming and secure knowledge retrieval—allows enterprises to scale agentic AI at the edge without the burden of managing costly, inefficient infrastructure.
Now is an ideal time to lay the groundwork for long-term AI success. Thoughtful infrastructure investments today will set enterprises up for long-term success and future innovations.