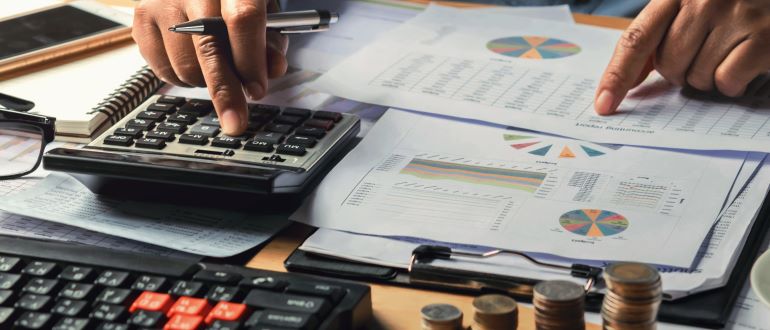
All security breaches are damaging, but very few breaches hit as hard as those affecting financial institutions.
Financial institutions hold extremely sensitive — and therefore valuable — information for millions of people, and though they are willing to pay to protect it, these breaches still happen. In a recent Integris report, 86% of surveyed bank executives said that “cybersecurity was a top concern and their biggest area of budget increases.” Given that 88% of those same executives also plan to increase their IT spending by at least 10%, this should help cybersecurity professionals feel a little better about their 2025 prospects.
There’s just one problem though. Even as these banks increase their spendings on cybersecurity, they are also increasingly adopting artificial intelligence (AI). 72% of banks have already incorporated AI, and according to IBM, “60% of banking CEOs surveyed acknowledge they must accept some level of risk to harness automation advantages and enhance competitiveness.” As a cybersecurity professional, any executive willing to “accept the risks” of such a powerful – and relatively new – technology has me right back to worrying.
For my fellow professionals at banks and other financial institutions who may receive extra budget this year, it’s time to consider how best to allow your organization to reap the benefits of AI in a relatively safe manner.
Building a Culture of AI Security
Although the majority of banks have adopted AI, far fewer of them have a robust AI use policy or training materials. Speaking to American Banker, Benesch Law partner Aslam Rawoof said, “The AI policies that I have seen at most banks are pretty immature.” Meanwhile, a McKinsey survey found only 31% of organizations with $500M+ in revenue had put together employee training modules on using GenAI.
Considering that many employees across industries, banking included, have started to use generative AI, this could quickly balloon into a problem. SAS research reports that “six in 10 [banking leaders] said they have deployed at least one GenAI use case to date – the highest of any industry.” But unsanctioned use is also common, with Software AG finding about half of all knowledge workers use their own AI tools.
This may make you think that employees will ignore your written policy even if you have one. Instead, advocate for the organization to adopt one enterprise model overall in addition to creating a policy.
Making the policy clear and model easy to access encourage employees to follow these rules. On top of that, further clarifying why these policies exist reinforces that organizational security is everyone’s responsibility. Security should be a fundamental part of your culture, especially as a repository of valuable financial information.
To illustrate the dangers of putting sensitive data into a large language model (LLM), one needs to only look at how much big tech companies are willing to pay those who find ways to break them. Microsoft put together a $10,000 prize pool for a prompt injection challenge in a sample LLM-powered email client. Another hacker won almost $50,000 after 482 attempts to manipulate an AI chatbot.
Addressing Side-Channel Attack Vulnerabilities
We already know that LLMs and the models connected to them are extremely susceptible to side-channel attacks. Redditors positively reviewing certain restaurants so that tourists are more likely to see them in an AI search and visit there is a relatively benign form of this attack.
The problem arises when a bad actor takes advantage of this vulnerability. For example, they can create a fake repository nearly identical to a legitimate one, then begin posting about it on sites like Reddit or other sources of model training data to make it more likely to be used. All of the code a model then generates will be immediately compromised, and the hacker can access any data or systems the code is deployed on.
This isn’t a new tactic by any stretch. I once presented on a similar method to compromise continuous integration and continuous delivery servers; the issue was so widespread that GitHub temporarily restricted searching for these servers as a stop-gap.
Although using tactics like GitHub’s can be effective in the short term, we don’t have a way to completely prevent side-channel attacks from compromising AI right now. For example, using a model trained on data scraped from the internet probably offers the most competitive edge, but automatically runs the risk of compromised code being generated. If you’re in a position to curate what data sources a model uses, you can filter out this kind of manipulation before it becomes poisoned code.
As a security team, using that increased budget to put in robust, scalable and high-quality testing for all code can also help protect your organization from this insidious attack method. That includes using tools designed to scan for malware in repositories or surface other parts of code that could compromise your servers.
Advocating for Continuous Investment
As a cybersecurity professional, I’ve been in a number of meetings where I need to explain to executives why they need to continue allocating a portion of the budget to something that doesn’t always produce visible results or returns. After all, in cybersecurity, our job is to prevent these attacks from happening, and if we do our jobs right, we may accidentally lull executives into a false sense of security.
Although the current increased investment can help your team patch today’s problems, none of us can predict tomorrow’s issues. However, the very technology you’re trying to protect your organization from may become an asset when you advocate for next fiscal year’s budget.
AI has its uses in cybersecurity, too. It can automate some of the most time-consuming tasks, such as deduplication. With automation in place, your team can shift focus to strategic initiatives, improving your overall security posture. If your organization is already investing heavily in AI, discussing its security applications could help make your case for cybersecurity investment overall.
Perhaps the best argument for cybersecurity investment, however, is to look at what breaches have cost other financial institutions. Not even major companies like Equifax and Capital One are completely immune. Breaches are only becoming more common: Since the SEC launched new cybersecurity disclosure rules, disclosures are up 60% overall. And the costs of breaches are also going up; the SEC issued over $63 million in fines to 12 firms in January.
But more importantly, the true cost of a data breach is impossible to put in numbers, no matter how many figures. When your institution is breached, you lose your customers’ trust, the most precious currency of them all. As business becomes more automated and even more competitive, protecting your institution from AI’s vulnerabilities may just be priceless.