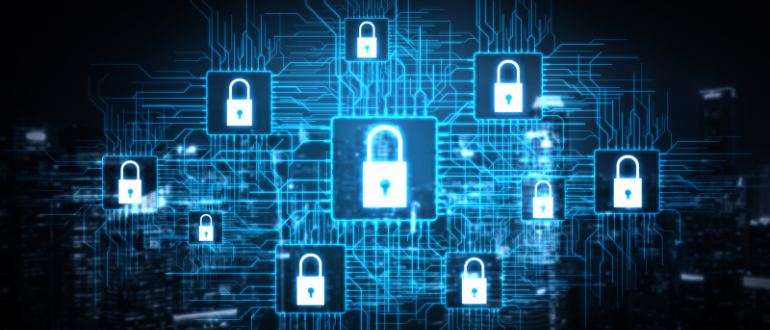
Probabilistic edicts emerging from black box systems might make the masses happy. But for enterprise security and compliance, standard artificial intelligence (AI) applications built on large language models (LLMs) don’t cut the mustard.
While security is never perfect, a bare minimum for building truly trusted, accountable systems requires provenance and traceability. These principles are becoming increasingly imperative for LLMs and all forms of AI. Provenance and traceability provide a clear lineage of data and decision-making processes, ensuring that AI systems are trustworthy, explainable and compliant with ethical standards. In the very near future, all AI compliance will likely hinge on the ability of applications and APIs powered by machine learning to clearly demonstrate the source and pathway of outputs.
What Does Provenance and Traceability Really Mean?
Provenance refers to the documented history and lineage of data, tracing its origins, transformations and movement throughout its lifecycle. In the context of AI, provenance is vital for ensuring that the data used to train models is authentic and trustworthy. This is particularly important for LLMs, where the quality and integrity of the training data directly influence the model’s behavior and outputs. Provenance can also apply to the outputs of models. Knowing what image, video, text or other outputs emitted from which specific AI system is critical to establishing true accountability and traceability.
While provenance is the starting point for traceability, this facet involves understanding where AI data comes from, how AI models are built and what happens when AI makes decisions. True traceability can also follow the trajectory of thinking through a model as it progresses down a mixture-of-experts or chain-of-thought. We see a visual version of this when DeepSeek R1 is “thinking” on the screen. For compliance and security, traceability provides a clear, auditable path that reveals the story behind an AI’s actions, similar to supply chain transparency in manufacturing or applying spectroscopy to understand the likely sources of isotopes.
The Role of Watermarking and Steganography
To enhance provenance and traceability, some AI solutions are leveraging watermarking and steganography. These techniques involve embedding unique identifiers into AI-generated content, allowing organizations to track its origin and authenticity. Unlike visible watermarks, steganographic methods hide these identifiers in plain sight, making them resilient against tampering and unauthorized sharing.
For instance, advanced watermarking solutions like those offered by Steg.AI and EchoMark use deep learning models to embed watermarks that are both invisible and robust against common tampering methods. These watermarks serve as digital fingerprints, enabling organizations to identify and address unauthorized content distribution, even if the content is altered or shared through screenshots or reformatting.
Integrating OpenTelemetry for Enhanced Observability
To further bolster the integrity of AI systems, OpenTelemetry (OTel) plays a crucial role. OTel is an open standard observability framework that standardizes the collection and analysis of telemetry data, including traces, metrics and logs. By integrating OTel with AI watermarking and steganography, organizations can achieve comprehensive visibility into AI operations.
The benefit of open standards is a larger ecosystem of tools, so a further benefit is the ease and portability of turning the data into insights. OTel enables the instrumentation of AI applications to emit telemetry data that can be analyzed for performance optimization, anomaly detection and compliance monitoring. This integration ensures that AI systems are not only accountable but also performant and secure. For example, using OTel to monitor AI-generated content allows organizations to track how watermarked content is used, ensuring that any unauthorized distribution can be quickly identified and addressed. OTel is becoming the standard for monitoring all metrics across clouds in cloud-native applications and Kubernetes. This is extending into AI applications, because most AI application training and inference are running in Kubernetes clusters.
Future Directions
As more and more enterprises weave AI capabilities into their technology fabric — both customer-facing and back-end — the importance of trust will grow. AI compliance regulations and insurance requirements are already forcing enterprises to think deeply about provenance and traceability. There are numerous methods that companies are proposing and pursuing to deliver trusted AI that is observable and traceable. Many of them, frankly, remain untested and are sufficiently novel to cause concern should they be rolled out on critical production systems.