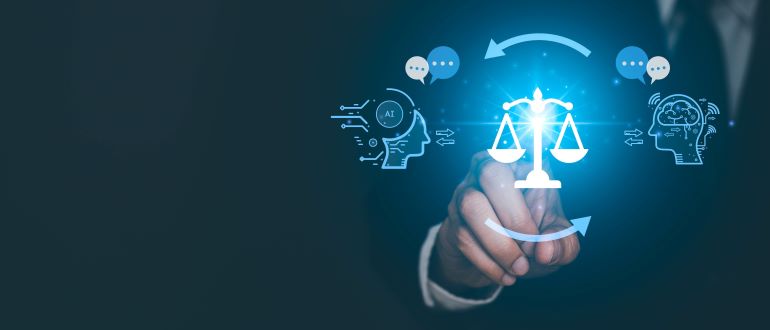
GMI Cloud recently released a new product, the GMI Cloud Inference Engine, providing cloud-based AI inference at scale. The world of AI continues to evolve at a rush, but this particular offer may be a sign that we’ve reached an interesting inflection point: We may finally be maturing beyond “bigger is better” phase of AI infrastructure into the self-evident business benefits it promises: growth and revenue-generation.
The Two Sides of AI
This shift highlights a still-too-often-overlooked reality of AI implementations: Training and inference are fundamentally different beasts that require fundamentally different approaches. For those just getting familiar with AI deployment cycles, it’s necessary to clarify these two distinct elements.
Training is the method for creating the AI: The compute-intensive, discrete process where models are developed and learn from datasets that are usually vast (even by today’s data-heavy standards). It is training that makes headlines; when companies announce they’ve trained models on trillions of parameters using thousands of GPUs, it’s the kind of flashy, impressive news that tech journalists love to write about, and we love to read.
But it is inference—the AI you interact with—that is the part that produces value, when trained models are put to work in the real world. It’s quite different, and not only because it’s online by definition. It has to run more or less continuously across pretty much the full gamut of production environments; and critically, has a similarly wide variety of production requirements, whether they be performance, power draw, cost, security, compliance, or other factors related to business or organizational goals or needs.
These elements couldn’t be more different in their technical strategies. Yet until recently, many organizations have treated them as if they were the same at the strategic level, throwing similar resources at both problems.
Successful Inference = Successful AI
For years, AI practitioners have emphasized that inference isn’t an afterthought—it requires its own dedicated strategy and technology stack. GMI Cloud’s new Inference Engine is intended to align with this philosophy, taking a more mature approach to successful AI than just raw power. Success in AI is about precision, flexibility and alignment with business goals.
The operational characteristics in inference can have a large impact on business value: think availability, geographic footprint, response times, business continuity, security posture, total cost and cost model. There are more factors too, that no one but the organization itself, knowing its own scenario and goals; altogether these determine whether an AI implementation actually delivers on its promises or becomes an expensive technical test that must be rescaled and adjusted to provide practical value.
While training demands brute-force computation, inference is where the right balance of performance, cost, and responsiveness drive success in production implementations. GMI Cloud’s approach is to allow customers to optimize inference to fit the needs of the entire business scenario, not just the model’s technical requirements. In practice this means flexible instance types, region-aware deployments, and performance-tuned presets for a good variety of popular LLMs. The company also offers customized solutions with the Inference Engine, eschewing a one-size-fits-all approach.
Starting with the Business, Not the Technology
Perhaps the most illustrative aspect of GMI Cloud’s approach is that, according to the company, it begins not with technological capabilities but with business outcomes. This is practically a trope in any analyst’s piece on how to formulate any particular technology strategy. But it’s not often-enough connected all the way down to the infrastructure design.
When we talk about AI, the conversation too often centers solely around model behavior—how accurate it is, how human-like its responses are, or how well it performs on benchmark tests. These factors matter, of course, but they’re just half (maybe less) of the puzzle. As noted above, operational characteristics in inference, such as availability, cost, security, and latency, significantly impact business value and must be properly balanced to determine the true success of AI.
In short: not every aspect of AI needs to be maximized. Instead, balance and optimize your inference stack based on your priorities, especially the business outcome you expected from the AI in the first place. A customer service chatbot might prioritize low latency and high availability over accuracy and transparency, for example; while a medical diagnosis tool might make the opposite trade-off.
Tailoring Infrastructure to Purpose
GMI Cloud’s Inference Engine’s approach, according to the company, is to allow customers to tailor the infrastructure their AI runs on based on their full set of requirements, not just performance benchmarks. This would go beyond the all-too-typical approach of providing infrastructure tuned for specific foundational models. Even organizations using the same AI model can have wildly different priorities based on the use case, which should be comprehensively understood including customer expectations, regulatory environment, business model, and so forth.
For example, a global financial institution might prioritize region-specific deployments to maintain data sovereignty and compliance, while a gaming company might focus on minimizing latency for real-time player interactions. One company might have to optimize cost above all else, while another might prioritize reliability and integration with existing systems.
Evolution in AI Deployment
A holistic, business-aligned approach to AI tactics is the next logical step for all of us in AI. We need to move quickly beyond buying up AI resources willy-nilly, and from asking “Can we make this work at all?” and “Can we make this work at scale?” to “How do we make this work that clearly and measurably support our business goals?”
It’s a sign of a maturing market where the technology itself is becoming less of a differentiator than how well it’s applied to specific scenarios at each individual organization. Some parts of AI, after all, will surely become more commoditized through natural market action, making the competitive advantage increasingly founded on how effectively organizations align their AI, from infrastructure to UX, with their unique setting.
The focus is shifting beyond just teaching computers to think. Organizations are working on optimizing entire ecosystems for AI-driven decision-making, ensuring maximum output while operating within resource constraints. There are a number of companies making strides in this area; GMI Cloud is addressing the challenge of balancing software improvements with the right infrastructure choices to achieve efficient, scalable inference.
As we look to the future of AI deployment, the distinction between implementing training and implementing inference will likely become even more pronounced.
For organizations implementing AI today, the lesson is clear: inference deserves its own focus and strategy and is hardly “training, but in production.” And inference strategies start with business outcomes and require technical implementations that support all of them to the greatest degree possible. GMI Cloud’s Inference Engine is a good example of how the industry is evolving to meet this need.