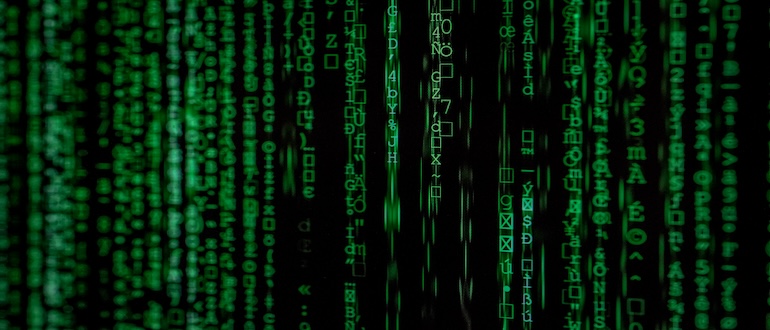
The same reality seen from different perspectives can appear vastly different.
A mathematician looking at the explosive growth of generative artificial intelligence (AI) may be happy to see the technology finally catching up with the theory. A business owner looking at the same technology however may see an entirely new and untested tool that may or may not be transformational.
Both realities are valid, yet neither vision is complete nor predicts what the generative AI wave will bring.
Generative AI is undoubtedly bringing business value to many organizations, yet there are many unanswered questions about ethics, security and the future of the technology. We are still in the early innings of AI; the game will play on for a few years.
ChatGPT, the first widely known generative AI application, employed a chat-based interface to create the impression of conversing with a knowledgeable human. Other tools soon followed, including Stable Diffusion for image generation, and Sora for AI-generated video.
These applications utilize neural networks— a concept that originated in the 1940s— and are powered by technological advancements that enable more human-like generative capabilities.
Smaller neural networks have provided predictive AI since the 1990s by recognizing patterns in data to predict future outcomes, such as weather and crop forecasts and financial modeling.
Neural networks are trained by learning the patterns in existing datasets, producing a set of parameters or weights. New data is then sent to the model with those parameters; the model applies the relevant patterns to produce an output.
Predictive AI models have hundreds or thousands of parameters, requiring a small amount of input data and computing power to create a result. Generative models have billions of parameters, requiring vastly more data processing to yield an answer. The massive capability growth in graphics processing units (GPUs) has enabled the expansion of model size, training with staggering amounts of data and inference with billions of parameters.
Although predictive AI is used everywhere in business, it is often invisible within features where past data is used to predict future performance. Generative AI is much more visible in those products.
As Ray Lucchesi, IT consultant and contractor, said at the Delegate Roundtable at the AI Field Day event earlier this year, “These things are taking off; it’s a different world.”
There is far more change because GenAI is much earlier in the adoption cycle. New foundation models are being developed, and older ones are discarded; even the benchmarks used to evaluate models are rapidly retired and replaced with newer benchmarks.
Andy Banta, data storage veteran, doesn’t pointed out that generative AI is not mature: “We are being the alpha test of AI.”
Talking about AI as if it were a human and could reason or knowingly create is a common fallacy. Current generative AI apps, such as ChatGPT and Google Gemini, do not know the meaning of the questions we ask or the answers they provide. Neural networks work only with numbers; words are transformed into numbers to pass into the neural network. The massive models used in generative AI represent patterns from the numbers representing training data and are used to create patterns of numbers as output data. These large language models (LLMs) know the context of everything but do not know the meaning of anything.
Hallucinations occur when the LLM generates an output that is coherent but false. For example, a Google AI recommended putting glue on a pizza, complete with a recommendation of the amount and brand of glue to use.
There are many unanswered non-functional questions about generative AI. Generative AI models require vast amounts of training data; the parameters represent that data. The most common method of gathering training data is retrieving publicly available information from internet sites. Does posting on the internet imply consent for others to use your content? Should the AI application credit the original authors when it outputs a derivative? There have been stories of leading AI companies knowingly using data they were not entitled to access to train their models.
The output too can have ethical problems; biased input data will produce biased output, as shown in a study on Google Bard in 2023. There have also been very public instances of generative AI models being poisoned during training or post-deployment. These issues are symptoms of a field where innovation is rapid. There are no industry standards yet for building and using the technology.
Generative AI has undergone massive innovation and will continue for years. It offers enormous benefits to businesses, like predictive AI. However, we need to see more standardized approaches to ethics around training data and AI safety in general. AI is helping to make complex information more accessible and complex systems simpler to manage. Over time, the technology will become a feature that makes applications work better and helps people be more productive. But that transformation will probably take a few more years.